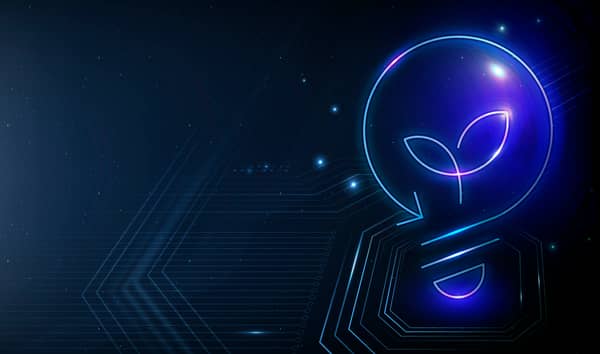
The Impact of Artificial Intelligence on Energy Saving in Telecoms
2024-07-09
Now more than ever, companies are seeking productivity gains through AI automation, leveraging artificial intelligence to streamline processes. Central to this evolution is Machine Learning Operations (MLOps), a discipline that ensures AI projects transcend theoretical models to become integral, functioning elements of business processes. This article is meant for organizations navigating the complexities of successful implementation of AI projects through MLOps frameworks. It covers crucial aspects, including development considerations, and deployment strategies emphasizing the pivotal role of Machine Learning Operations (MLOps) in unlocking AI's transformative potential for enhanced productivity and efficiency.
In today's fast-paced business landscape, companies are constantly seeking ways to enhance productivity, efficiency, and profitability. One technology that has gained significant attention in recent years is AI automation, which refers to the use of artificial intelligence to automate repetitive tasks, streamline processes, and improve decision-making. As businesses increasingly lean on AI to streamline operations, enhance customer experiences, and innovate products, the demand for a robust framework to manage these AI systems has never been higher. MLOps addresses this need by bridging the gap between machine learning model development and operational deployment, ensuring AI projects are not only successfully launched but also continuously optimized and aligned with business objectives.
Investing in AI automation can offer numerous benefits to businesses, including increased productivity, cost savings, and improved customer experience. However, it can be a difficult task with complex decisions to be taken and many factors to consider. As AI continues to reshape industries, businesses are eager to tap into its potential, but implementing a successful AI automation strategy is hardly possible without implementing a core MLOps (Machine Learning Operations) framework. MLOps is not just about operational efficiency; it's also about strategic alignment. It helps bridge the gap between creating AI models and using them in real-world situations streamlining the entire process, from development to deployment and ongoing maintenance.
MLOps takes intelligent process automation (IPA) to the next level. While IPA automates tasks, MLOps ensures the ongoing success of AI models powering that automation. Think of MLOps as the control center for your IPA initiatives. It facilitates seamless integration with existing systems and processes, working hand-in-hand with your IT team.
But MLOps doesn't stop at deployment. It empowers you to scale IPA effectively. A robust MLOps framework allows for rapid experimentation, deployment, and management of multiple AI models across various business areas and data sets. This enables you to gradually scale intelligent process automation, minimizing disruption while maximizing the benefits of AI technology.
Figure 1 – MLOps Life Cycle
The significance of embracing MLOps has never been more profound than nowadays, since it is crucial for businesses looking to stay competitive in the digital age. By providing a framework for scalable, repeatable, and reliable AI model deployment, MLOps enables businesses to integrate AI solutions seamlessly into their existing ecosystems, ensuring these technologies contribute directly to achieving strategic goals. Companies that have embraced MLOps report not only faster time-to-market for AI solutions but also improved performance, scalability, and return on investment. These case studies serve as compelling evidence of the tangible benefits MLOps brings to organizations committed to leveraging AI for long-term success. According to MarketsandMarkets, the MLOps market is projected to witness substantial growth from $1.1 Billion reported back in 2022 to $5.9 Billion by 2027. The substantial increase indicates a growing demand for MLOps solutions and services as organizations continue to prioritize efficient machine learning operations.
Imagine data as the new oil. MLOps acts as the refinery, ensuring this precious resource is refined and utilized effectively. It standardizes the machine learning lifecycle, making it predictable and scalable. It takes charge of every step, from data collection and cleaning to model training, validation, deployment, and monitoring, showcasing the comprehensive oversight that IPA, like MLOps, provides. MLOps smooths the path for these models to seamlessly transition into production, driving business value and creating a competitive edge.
Figure 2 – MLOps Market Size Evolution (Marketsandmarkets)
Selecting appropriate MLOps tools is pivotal for the efficacy of AI projects. These tools facilitate various stages of AI model lifecycle management, including development, deployment, monitoring, and maintenance. The right MLOps platform can dramatically enhance the efficiency of these processes, ensuring that AI solutions deliver on their promise of driving business value. On the other hand, the wrong tools can lead to wasted resources, delayed projects, and suboptimal outcomes.
There are several factors to consider when choosing MLOps tools. These include the tool's compatibility with your existing systems, its scalability to accommodate future growth, its ability to integrate with other tools in your tech stack and its support for the machine learning frameworks and languages used. One such tool should be designed for domain experts, not developers or data scientists, facilitating the creation of complex automation utilities within any industry, delivering a quality product that is stable, scalable, and easy to maintain. This MLOps tool should provide a simple and flexible framework to create end-to-end automation applications relying on a series of UI-based utilities that facilitate transferring ideas into real products with no programming required.
Furthermore, you should consider the tool's ease of use, the quality of its documentation and community support, and the reputation of its vendor. A good MLOps tool not only meets your current needs but also grows with you, adapting to your evolving requirements and challenges.
MLOps offers a systematic method for quickly creating, deploying, observing, and updating AI/ML applications. Effective MLOps workflows equip data scientists or SMEs with the necessary resources to constantly oversee AI models and adjust to fluctuations in their data.
Yet, the engineering-centric perspective of MLOps frequently reduces AI model creation, deployment, supervision, and upkeep to being perceived as merely another form of technology. Organizations adopting this viewpoint, expecting data science teams to deduce how AI ought to adjust to organizational changes, frequently find the outcomes unsatisfactory.
Choosing the right vendor can minimize this risk, as the approach taken can be extremely different from one vendor to another. The key to choosing the right vendor with the appropriate strategy is to always have the true business value as the pillar of any MLOps project. Many businesses struggle with bridging the gap between model development and actual business value creation. The strategy for AI and its implementation should focus more on aligning with a company's overall business strategy than on refining models to perfection.
Of course, to evaluate AI vendors effectively, you also need to consider factors such as their track record, domain expertise, technology stack, and scalability. However, the most essential part is to assess the vendor's ability to understand your business requirements and align their AI solutions accordingly. Additionally, conducting a proof of concept (POC) or pilot project can help you assess the vendor's capabilities firsthand before committing to a long-term partnership.
Tupl stands out as an excellent AI vendor for businesses seeking to automate their operations and enhance their productivity. With its deep domain expertise, comprehensive technology stack, and commitment to solution customization, Tupl provides businesses with flexible and tailored AI solutions that drive efficiency, innovation, and sustainable growth. Tupl's collaborative approach, emphasis on POCs, and seamless integration ensure that businesses fully engage in the AI journey and achieve their desired outcomes.
Tupl is a leading provider of AI solutions for the telecommunications industry and is growing in the manufacturing and agricultural sectors. Its approach to AI development and deployment offers valuable insights for businesses looking to invest in AI automation.
Tupl’s AI solutions are powered by TuplOS, a pragmatic MLOps platform designed for domain experts, not developers or data scientists, embodying the principles of no-code development. TuplOS facilitates the creation of complex automation utilities within any industry, delivering a quality product that is stable, scalable, and easy to maintain.
A key advantage of Tupl’s solutions is that they don’t require vast amounts of data. Instead, they utilize all available and relevant network and customer data to identify the most probable root cause of customer issues to then provide recommendations in natural language.
In terms of deployment, Tupl ensures a secure and seamless integration of AI solutions into existing systems, providing ongoing support and maintenance to optimize the performance of AI models and ensure a positive user experience.
By partnering with Tupl, telecom operators have been able to automate customer support processes, resulting in a 90% reduction in tickets and an increase of over 5% in First Call Resolution (FCR). Network performance has improved due to a 90% reduction in manual effort, leading to 90% more accuracy. Furthermore, overall operational efficiency has been enhanced, with 100% consistency across all operations.
As AI technologies continue to evolve, so will the methodologies and tools associated with MLOps. The future promises more advanced AI capabilities, necessitating equally sophisticated MLOps solutions to manage these technologies effectively. Staying abreast of these developments will be crucial for businesses aiming to maintain a competitive edge in the AI-driven landscape.
MLOps stands at the heart of successful AI automation, offering the tools and processes needed to transition AI from concept to core business driver. As companies look to leverage AI for growth and efficiency, understanding and investing in MLOps will be key to unlocking these technologies' full potential. Embracing MLOps means not just participating in the AI revolution but leading it, armed with the capabilities to innovate, scale, and thrive in the digital age.
Rapidly evolving technologies are also starting to shape the future of MLOPs: an emerging trend is the rise of generative AI, which refers to AI systems that can generate new content, such as text, images, and videos. Generative AI (such as the famous Chat GPT) has the potential to revolutionize AI Automation and MLOps processes quickly. As a matter of fact, companies like Tupl have already started integration of the Gen AI technology with their solution: they just presented the first integration of GenAI with AI Care, a solution that automates root cause analysis and ticket resolution for telecom operators' customer care agents. With this integration, the AI Care users - mainly care agents in first line and network engineers in second line -have a new way to communicate with the AI Care tool, with a friendlier interface that allows them to ask further questions to investigate the first root cause analysis and context data provided by AI Care. In the typical Gen AI format, the care agents can interactively investigate by asking questions and receiving answers in natural everyday language. And this is just the beginning, as Tupl is already working on this integration with other Tupl solutions such as Network Advisor.
While investing in AI automation can propel businesses towards greater efficiency, productivity, and competitiveness, bridging the gap between development and real-world impact requires MLOps. This crucial element streamlines the entire lifecycle, ensuring efficient experimentation, deployment, and management of your AI models. Think of it as the refinery for your data, transforming it into valuable business solutions.
Companies like Tupl have demonstrated the transformative potential of AI automation. By choosing the right MLOps tools like TuplOS, designed specifically for domain experts, you can unlock its power with ease. Start with a clear AI strategy aligned with your business goals and leverage Tupl's expertise to implement and scale your initiatives seamlessly.
AI automation isn't just about staying competitive; it's about thriving in a data-driven future. Embracing MLOps, coupled with the right tools, unlocks a world of possibilities.